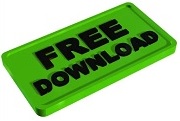
The fitted ANOVA is passed to TukeyHD() as an input. We can compute Tukey HSD (Tukey Honest Significant Differences, R function: TukeyHSD()) for doing numerous pairwise comparisons between the means of groups because the ANOVA test is significant. Multiple pairwise comparisons can be performed to see if the mean differences between certain pairs of groups are statistically significant. Multiple pairwise comparisons between group meansĪ significant p-value in an ANOVA test shows that some of the group means differ, but we don’t know which pairings of groups differ. Replace the plus symbol (+) with an asterisk (*) if you think these two variables will interact to create a synergistic effect. It is presumptively assumed that the two-factor variables are unrelated. The above-fitted model is not referred to as an additive model. These findings led us to anticipate that modifying the delivery technique (supp) or the vitamin C dose will have a major impact on the mean tooth length. The most important factor variable is dosage. We may deduce from the ANOVA table that both supp and dose are statistically significant. The columns F value and Pr(>F) in the output corresponding to the p-value of the test.
#ONE WAY ANOVA IN R STUDIO TRIAL#
The trial used 60 pigs who were given one of three vitamin C dose levels (0.5, 1, or 2 mg/day) via one of two administration routes (orange juice or ascorbic acid) (a form of vitamin C and coded as VC).Ī sample of the data is presented below, which includes tooth length measurements. It includes information from a study on the effects of vitamin C on tooth growth in Guinea pigs. We’ll use the built-in R data set ToothGrowth for this. When we have equal sample sizes within levels of our independent grouping levels, we call it a balanced design.

In R, create a two-way ANOVA test using balanced designs
#ONE WAY ANOVA IN R STUDIO HOW TO#
Like all ANOVA tests, two-way ANOVA assumes that the observations within each cell are normally distributed with equal variances.Īfter fitting ANOVA, we’ll teach you how to double-check these assumptions.
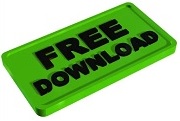